2025 Author: Leah Sherlock | [email protected]. Last modified: 2025-01-24 17:46
Previously known only from science fiction books, the term neural network in recent years has gradually and imperceptibly entered public life as an integral part of the latest scientific developments. Of course, for quite a long time, people involved in the gaming industry have known that this is a neural network. But nowadays the term is found by everyone, it is known and understood by the broad masses. Undoubtedly, this indicates that science has become closer to real life, and new breakthroughs await us in the future. And yet, what is a neural network? Let's try to figure out the meaning of the word.
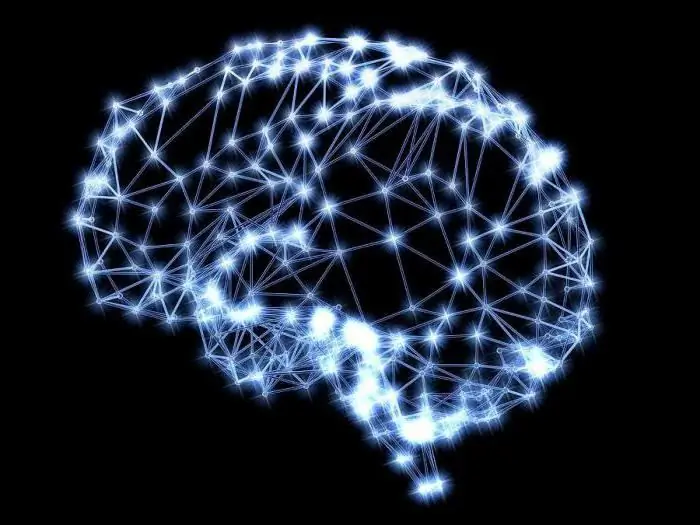
Present and future
In the old days, a neural network, Hort and spacewalkers were closely related concepts, because it was possible to meet artificial intelligence with abilities far superior to a simple machine only in a fantasy world that arises in the imagination of some authors. And yet, the trends are such that recently around an ordinary person in reality there are more and more of those objects that were previously mentioned only in science fiction literature. This allows us to say that even the most violent flight of fantasy, perhaps, sooner or later will find its equivalent in reality. Books about hits, neural networks alreadynow have more in common with reality than ten years ago, and who knows what will happen in another decade?
A neural network in modern realities is a technology that allows you to identify people, having only a photograph at your disposal. Artificial intelligence is quite capable of driving a car, can play and win a game of poker. Moreover, neural networks are new ways to make scientific discoveries, allowing you to resort to previously impossible computing capabilities. This gives unique chances for understanding the world today. However, only from news reports announcing the latest discoveries, it is rarely clear what a neural network is. Should this term be applied to a program, a machine, or a complex of servers?
General view
As you can see from the very term "neural network" (the photos presented in this article also make it possible to understand this) is a structure that was designed by analogy with the logic of the human brain. Of course, copying a completely biological structure of such a high level of complexity at the moment does not seem realistic, but scientists have already been able to noticeably come closer to solving the problem. Let's say that recently created neural networks are quite effective. Hort and other writers who published fantastic works hardly knew at the time of writing their works that science would be able to step so far ahead by this year.
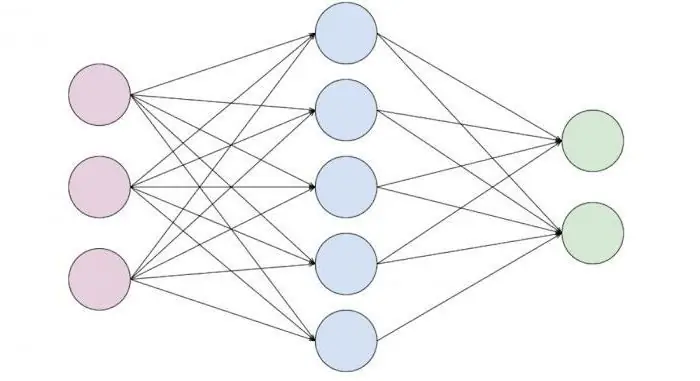
The peculiarity of the human brain is that it is a structure of numerous elements, between whichinformation is constantly transmitted through neurons. In fact, new neural networks are also similar structures, where electrical impulses provide the exchange of relevant data. In a word, just like in the human brain. And yet it is not clear: is there any difference from a conventional computer? After all, the machine, as you know, is also created from parts, the data between which are transferred by means of an electric current. In books about space, neural networks, everything usually looks enchanting - huge or tiny machines, at one glance at which the characters understand what they are dealing with. But in reality, the situation is different so far.
How is it built?
As you can see from the scientific papers on neural networks (“Spacewalkers”, unfortunately, do not belong to this category, no matter how fascinating they may be), the idea in the most progressive structure in the field of artificial intelligence, in creating a complex structure, the individual parts of which are very simple. In fact, drawing a parallel with humans, one can find a similarity: say, only one part of the brain of a mammal does not have great abilities, capabilities, and cannot provide intelligent behavior. But when it comes to a person as a whole, then such a creature calmly passes the test for the level of intelligence without any particular problems.
Despite these similarities, a similar approach to creating artificial intelligence was ostracized a few years ago. This can be seen both from scientific papers and from science fiction books about the neural network (“Spacewalkers” mentioned above, for example). By the way, to some extent even statementsCicero can be associated with the modern idea of neural networks: at one time, he rather caustically suggested that monkeys throw letters written on tokens into the air, so that sooner or later a meaningful text would form from them. And only the 21st century showed that such malice was completely unjustified. The neural network and science fiction went their separate ways: if you give an army of monkeys a lot of tokens, they will not only create a meaningful text, but also gain power over the world.
Strength is in unity, brother
As we learned from numerous experiments, training a neural network then leads to success when the object itself includes a huge number of elements. As scientists joke, in fact, a neural network can be assembled from anything, even from boxes of matches, since the main idea is a set of rules that the resulting community obeys. Usually the rules are quite simple, but they allow you to control the data processing process. In such a situation, a neuron (albeit an artificial one) will not be a device at all, not a complex structure or an incomprehensible system, but rather simple arithmetic operations, implemented with minimal energy consumption. Officially in science, artificial neurons are called "perceptrons". Neural networks (“Spacefalls” illustrate this well) should be much more complex in the view of some scientific authors, but modern science shows that simplicity also gives excellent results.
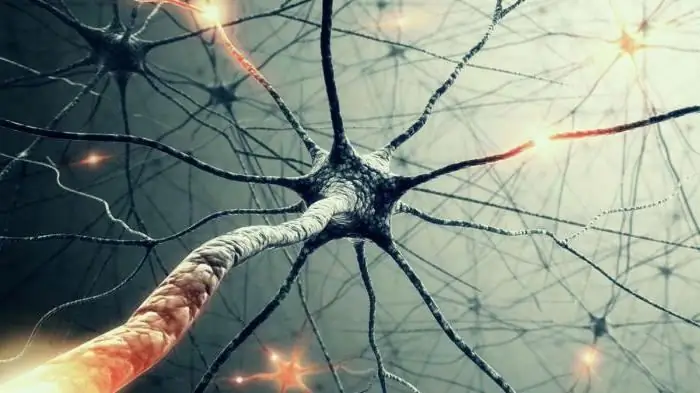
The operation of an artificial neuron is simple: numbers are input, the value for each is calculatedinformation block, the results are added up, the output is a unit or the value "-1". Did the reader ever want to be among the fallen? Neural networks work in a completely different way in reality, at least at the present time, therefore, when imagining yourself in a fantasy work, you should not forget about this. In fact, a modern person can work with artificial intelligence, for example, like this: you can show a picture, and the electronic system will answer the question “either - or”. Suppose that a person sets the coordinate system of one point and asks what is depicted - the earth or, say, the sky. After analyzing the information, the system gives an answer - quite possibly incorrect (depending on the perfection of the AI).
Thumbs up
As you can see from the logic of the modern neural network, each element of it is trying to guess the correct answer to the question asked to the system. In this case, there is little accuracy, the result is comparable to the result of tossing a coin. But the real scientific work begins when it comes time to train the neural network. Space, exploration of new worlds, insight into the essence of the physical laws of our universe (which modern scientists rely on using neural networks) will become open at the very moment when artificial intelligence will learn with much greater efficiency and effectiveness than a person.
The fact is that the person who asks the system a question knows the correct answer to it. So, you can write it in the information blocks of the program. A perceptron that gives the correct answer gains value, andhere the one who answered incorrectly loses it, receiving a fine. Each new program launch cycle differs from the previous one due to the change in value level. Returning to the previous example: sooner or later the program will learn to clearly distinguish between earth and space. Neural networks learn the more effectively, the more correctly the study program is drawn up - and its formation costs modern scientists a lot of effort. As part of the task set earlier: if the neural network is provided with another photo for analysis, it will probably not immediately be able to process it accurately, but, based on the data obtained during the training earlier, it will accurately figure out where the earth is, and where are the clouds, space or something something else.
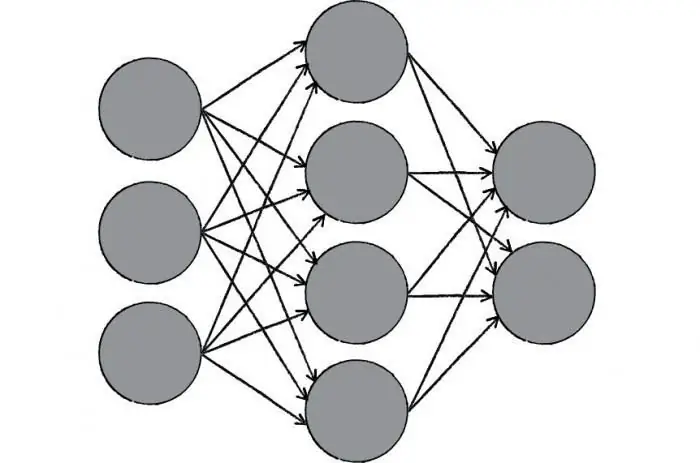
Applying an idea to reality
Of course, in reality, neural networks are much more complicated than those described above, although the principle itself remains the same. The main task of the elements from which the neural network is formed is to systematize numerical information. When combining an abundance of elements, the task becomes more complicated, since the input information may not be from outside, but from the perceptron, which has already done its job of systematization.
If we return to the task above, then inside the neural network you can come up with the following processes: one neuron distinguishes blue pixels from others, the other processes the coordinates, the third analyzes the data received by the first two, on the basis of which it decides whether the earth or the sky is in given point. Moreover, sorting into blue and other pixels can be entrusted to several neurons simultaneously, and the information they receive can be summarized. Those perceptrons that will givea better and more accurate result will receive a bonus in the form of a higher value at the end, and their results will be a priority when reprocessing any task. Of course, the neural network turns out to be extremely voluminous, and the information processed in it will be an unbearable mountain at all, but it will be possible to take into account and analyze errors and prevent them in the future. The largely neural-network-based implants found in many science fiction books work like this (unless, of course, the authors bother to think about how it works).
Historic milestones
It may surprise the layman, but the first neural networks appeared in 1958. This is due to the fact that the device of artificial neurons is similar to other computer elements, between which information is transmitted in the format of a binary number system. At the end of the sixties, a machine was invented, called the Mark I Perceptron, in which the principles of neural networks were implemented. This means that the first neural network appeared only a decade after the construction of the first computer.
The first neurons of the first neural network consisted of resistors, radio tubes (at that time, such a code that modern scientists could use had not yet been developed). Working with a neural network was the task of Frank Rosenblatt, who created a two-layer network. A screen with a resolution of 400 pixels was used to transmit external data to the network. The machine was soon able to recognize geometric shapes. This already suggested that, with the improvement of technical solutions, neural networks canlearn to read letters. And who knows what else?
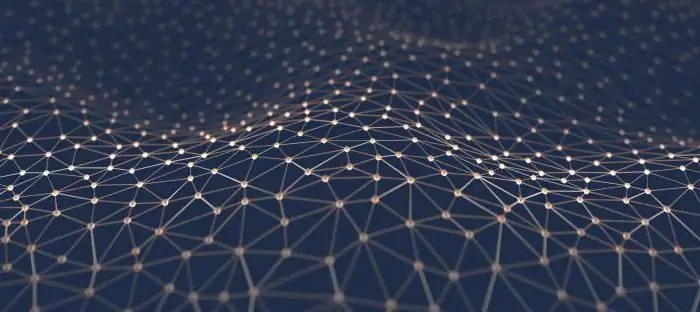
First neural network
As can be seen from history, Rosenblatt literally burned with his work, he was perfectly oriented in it, he was a specialist in neurophysiology. He was the author of a fascinating and popular university course in which anyone could understand how to implement the human brain in a technical embodiment. Even then, the scientific community hoped that soon there would be real opportunities to form intelligent robots capable of moving, speaking, and forming systems similar to themselves. Who knows, maybe these robots would go to colonize other planets?
Rosentblatt was an enthusiast, and you can understand him. Scientists believed that artificial intelligence could be realized if mathematical logic was fully embodied in a machine. At this point, the Turing test already existed, Asimov popularized the idea of robotics. The scientific community was convinced that the exploration of the Universe was a matter of time.
Skepticism justified
Already in the sixties there were scientists who argued with Rosenblatt and other great minds working on artificial intelligence. A fairly accurate idea of their logic of fabrications can be obtained from the publications of Marvin Minsky, well-known in his field. By the way, it is known that Isaac Asimov and Stanley Kubrick spoke highly of Minsky's abilities (Minsky helped him work on A Space Odyssey). Minsky was not against the creation of neural networks, about whichKubrick's film testifies, and as part of his scientific career, he was engaged in machine learning back in the fifties. Nevertheless, Minsky was categorical about erroneous opinions, criticizing hopes for which at that moment there was still no solid foundation. By the way, Marvin from the books of Douglas Adams is named after Minsky.
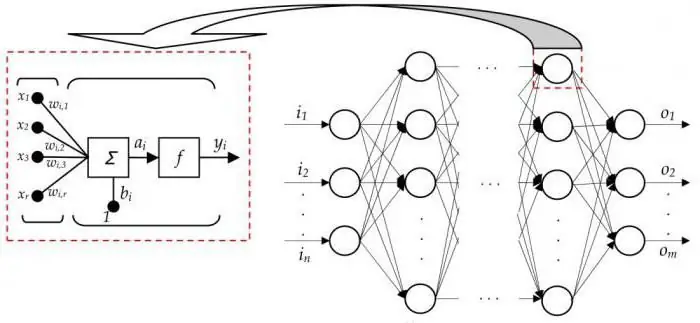
Criticism of neural networks and the approach of that time is systematized in the publication "Perceptron", dated 1969. It was this book that literally killed many people's interest in neural networks in the bud, because a scientist with an excellent reputation clearly showed that Mark the First had a number of flaws. Firstly, the presence of only two layers was clearly insufficient, and the machine could do too little, despite its gigantic size and huge energy consumption. The second point of criticism was devoted to the algorithms developed by Rosenblatt for network training. According to Minsky, information about errors was lost with a high probability, and the necessary layer simply did not receive the full amount of data for a correct analysis of the situation.
Things stopped
Despite the fact that Minsky's main idea was to point out mistakes to his colleagues in order to stimulate them to improve the development, the situation was different. Rosenblatt died in 1971, and there was no one to continue his work. During this period, the era of computers began, and this field of technology was moving forward with huge strides. The best minds in mathematics and computer science were employed in this sector, and artificial intelligence seemed like an unreasonable waste of forces and resources.
Neural networks have not attracted the attention of the scientific community for more than a decade. The turning point came when cyberpunk came into vogue. It was possible to find formulas by which errors can be calculated with high accuracy. In 1986, the problem formulated by Minsky already found a third solution (all three were developed by independent groups of scientists), and it was this discovery that prompted enthusiasts to explore a new field: work on neural networks became active again. However, the term perceptrons was quietly replaced by cognitive computing, got rid of experimental devices, began to use coding, using the most effective programming techniques. Just a few years, and neurons are already assembled into complex structures that can cope with quite serious tasks. Over time, it was possible, for example, to create programs for reading human handwriting. The first networks appeared capable of self-learning, that is, they independently found the correct answers, without a hint from the person controlling the computer. Neural networks have found their application in practice. For example, it is on them that programs that identify numbers on checks are used in banking structures in America.
Forward by leaps and bounds
In the 90s, it became clear that a key feature of neural networks that requires special attention of scientists is the ability to explore a given area in search of the right solution without prompting from a person. The program uses the trial and error method, on the basis of which it creates behavioral rules.
This period was marked by a surge of interestpublic to makeshift robots. Enthusiast designers from all over the world began to actively design their own robots capable of learning. In 1997, this marked the first truly serious success at the world level: for the first time, a computer beat the world's best chess player, Garry Kasparov. However, by the end of the nineties, scientists came to the conclusion that they had reached the ceiling, and artificial intelligence could not grow further. Moreover, a well-optimized algorithm is much more efficient than any neural network in solving the same problems. Some functions remained with neural networks, for example, the recognition of archival texts, but nothing more complicated was available. Basically, as modern scientists say, there was a lack of technical capabilities.
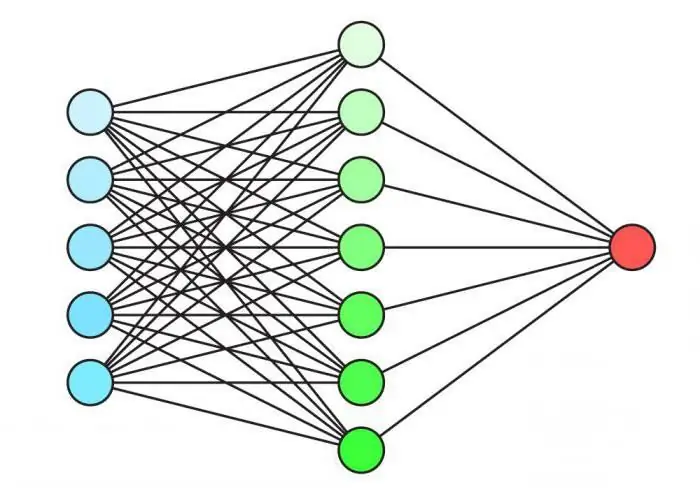
Our time
Neural networks today are a way to solve the most complex problems using the “solution will be found by itself” method. In fact, this is not connected with any scientific revolution, just modern scientists, the luminaries of the world of programming, have access to a powerful technique that allows them to put into practice what a person could only imagine before in general terms. Returning to Cicero's phrase about monkeys and tokens: if you assign someone to animals who will give them a reward for the correct phrase, they will not only create a meaningful text, but write a new "War and Peace", and not worse.
Neural networks of our days are in service with the largest companies working in the field of information technology. These are multilayer neural networks implemented through powerful servers,using the possibilities of the World Wide Web, the arrays of information accumulated over the past decades.
Recommended:
Cycle in literature - what is it? Meaning, definition and examples
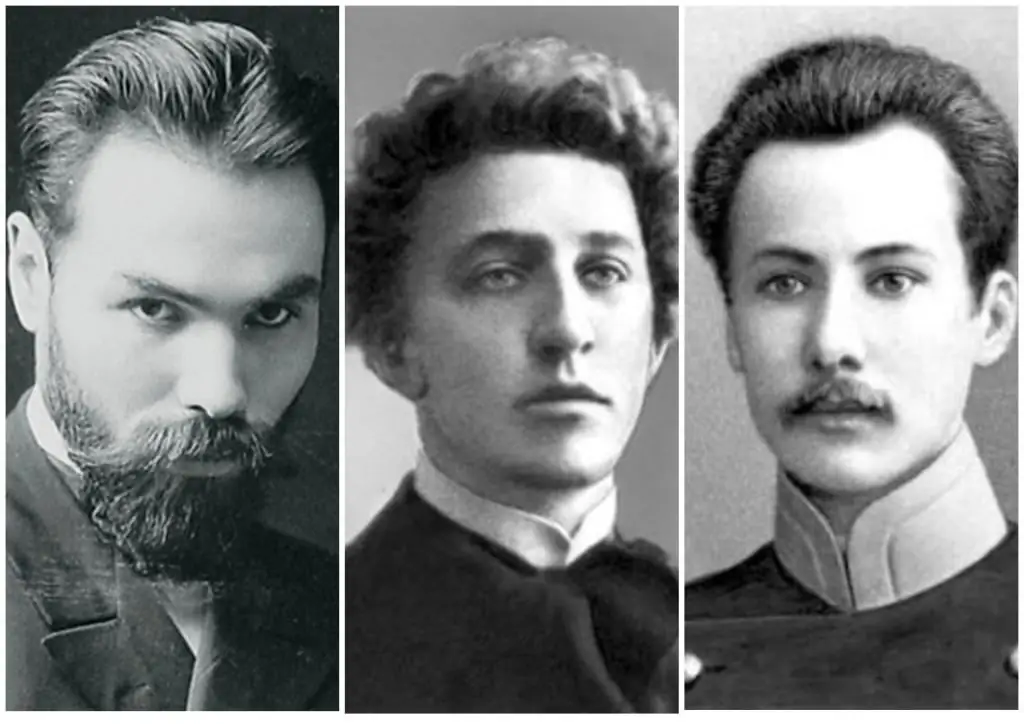
The established expression "cycle of works" does not always correspond to our ideas about what a literary cycle is. Is the storybook a cycle? And Pushkin's Belkin Tales? Amazing discoveries are given to us by philologists, studying the usual adventures of Dunno and other books
What is drama? Meaning and definition
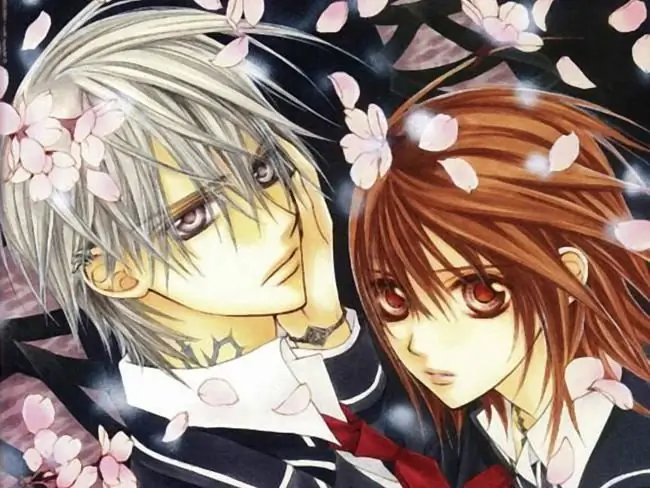
What is drama? This is a literary genre. Today, the term is used, as a rule, when it comes to a film that tells about tragic events. However, the word "drama" arose long ago, much earlier than the release of the film by the Lumiere brothers
Register in music is The meaning and definition of the word
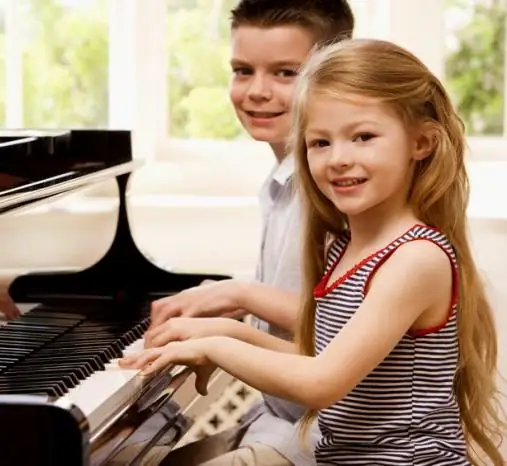
Register in music is, first of all, a series of sounds of a singing voice. It can also be a section of the range of any musical instruments. This is a short definition of register in music. And what is the meaning of this word? And how to explain the topic “Registers in Music” at the solfeggio lesson?
"A curious Barbara's nose was torn off at the market": the meaning and meaning of the saying
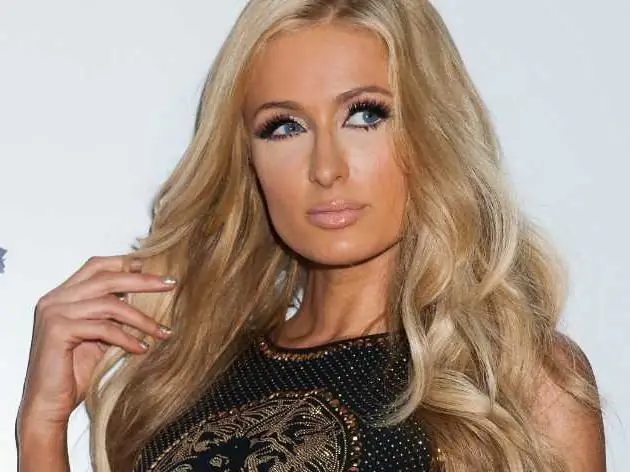
When we were children peeping at various interesting things, but not intended for the eyes of a child, our parents would catch us with the words: “The nose of the curious Varvara was torn off at the market”. And we understood what that meant, intuitively or consciously. In our article, we will deal with the meaning of this saying, and with whether it is good or bad to be curious
The Social Network biopic: plot, creators, actors ("The Social Network" 2010)
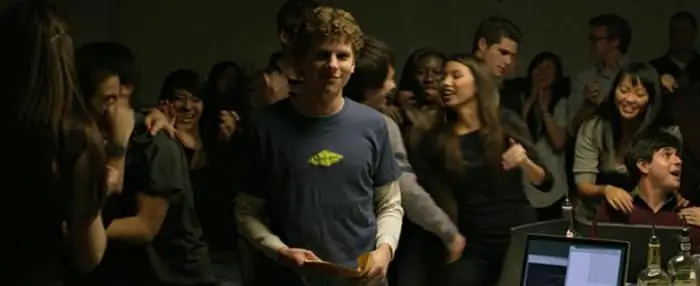
In 2010, director David Fincher presented to the audience a classic American success story with a modern interpretation, famous actors were involved in the work on the project. The Social Network is a canonical biopic, a film biography of the notorious Mark Zuckerberg